The `r` command in C++ provides an efficient way to read data from files, particularly useful for handling large datasets or input files in a streamlined manner.
Here's a simple code snippet demonstrating how to use the `r` command with file input:
#include <iostream>
#include <fstream>
#include <string>
int main() {
std::ifstream file("data.txt"); // Open the file in read mode
std::string line;
while (std::getline(file, line)) { // Read file line by line
std::cout << line << std::endl; // Output each line
}
file.close(); // Close the file
return 0;
}
Setting Up Your Environment
To start leveraging R cpp, you'll need to ensure your development environment is prepared. This includes installing necessary tools and setting up your code editor.
Requirements for using R cpp
First, make sure you have the following installed:
- R: The core language you will be interfacing with.
- Rtools: A collection of tools necessary for building R packages, useful for Windows users.
- Rcpp: The R package that acts as a bridge between R and C++.
You can easily check if R and Rcpp are correctly installed by running:
sessionInfo()
This command gives details about your R version and loaded packages, including Rcpp.
Configuring RStudio for R cpp development
RStudio is a fantastic IDE for R development and smoothly supports R cpp projects.
To get started, create a new project in RStudio:
- Go to File > New Project > New Directory.
- Choose R Package.
- Name your package and select a location.
You can now create your first Rcpp project here.
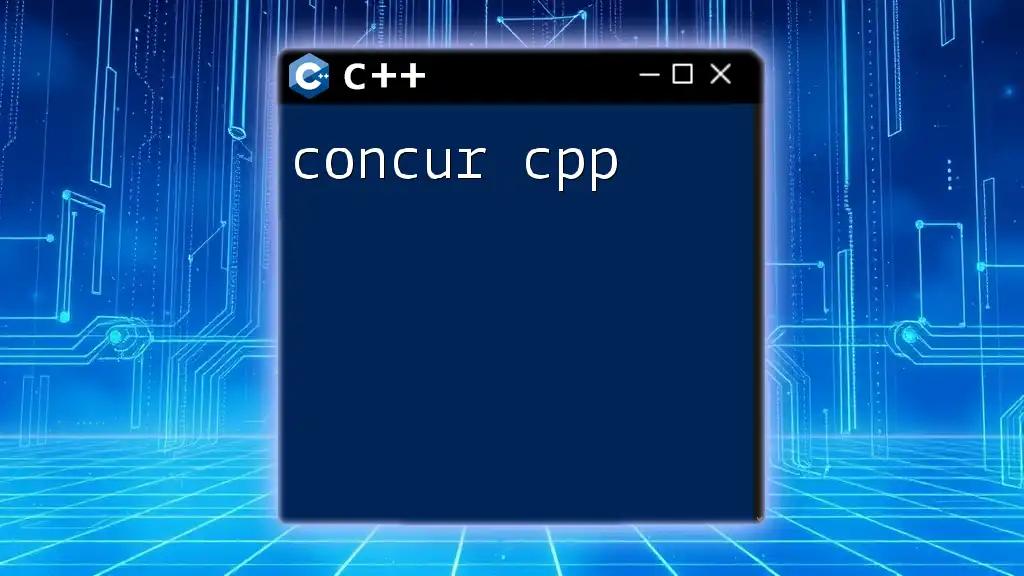
Basics of R cpp
Understanding the Rcpp package is crucial to successfully integrating C++ with R. Rcpp provides various functionalities, simplifying the process of calling C++ code and accessing C++ libraries from R directly.
Understanding the Rcpp package
Rcpp allows for the execution of C++ code within R, enhancing performance, especially when dealing with large datasets.
Key functions and features of Rcpp include:
- `.Call()`: A mechanism for calling C++ functions.
- `c++11` support: Enables modern C++ programming paradigms.
Writing your first Rcpp function
Here's how to define a simple C++ function and call it from R:
First, create a new file under the src directory of your R package and name it `example.cpp`. Then insert the following code:
#include <Rcpp.h>
using namespace Rcpp;
// [[Rcpp::export]]
double cpp_sum(NumericVector vec) {
return sum(vec);
}
The above code defines a function `cpp_sum` that calculates the sum of a numeric vector passed from R to C++.
To call this function in R, simply run:
library(Rcpp)
sourceCPP("src/example.cpp") # Adjust the path according to your directory structure
vec <- c(1, 2, 3, 4, 5)
result <- cpp_sum(vec)
print(result) # This should return 15
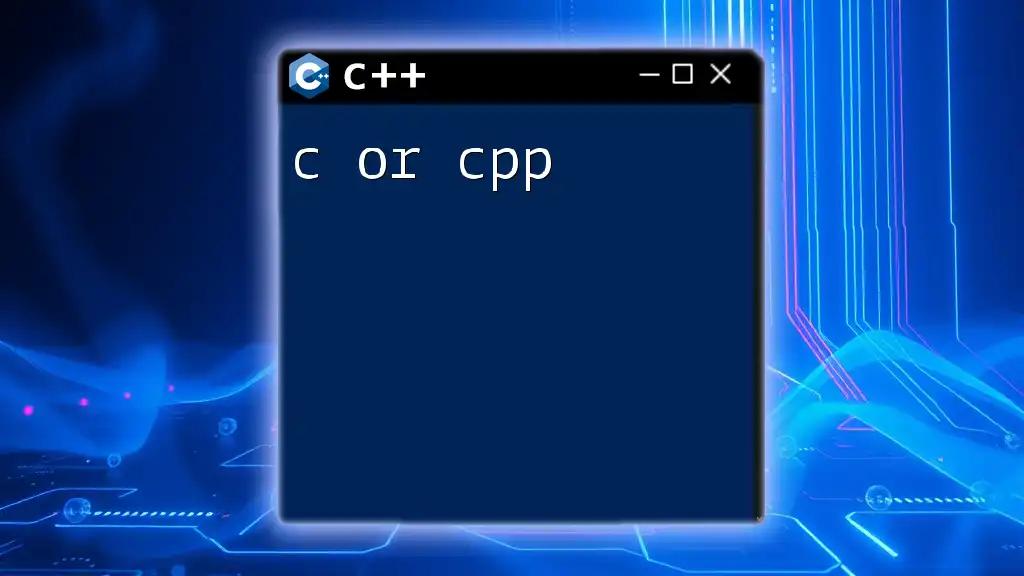
Enhancing R with C++: Data Types and Structures
To use R cpp effectively, understanding the data types and structures in C++ that correspond to R is essential.
C++ data types compatible with R
C++ offers several data types; knowing the equivalent in R will help streamline your coding process. Commonly used types include:
- `int`: Integer type
- `double`: Floating-point type
- `std::string`: String type
For instance, an R numeric vector corresponds to a `NumericVector` in C++.
Using STL containers in R cpp
The Standard Template Library (STL) provides flexible data structures. Accessing these from R cpp can significantly enhance your code's efficiency.
Here's an example of using STL's vector in R cpp:
#include <Rcpp.h>
#include <vector>
// [[Rcpp::export]]
double stl_sum(std::vector<double> vec) {
double total = 0.0;
for (double v : vec) {
total += v;
}
return total;
}
This function uses C++ STL vectors for input and sums them up. You can call `stl_sum` similarly to your prior function.
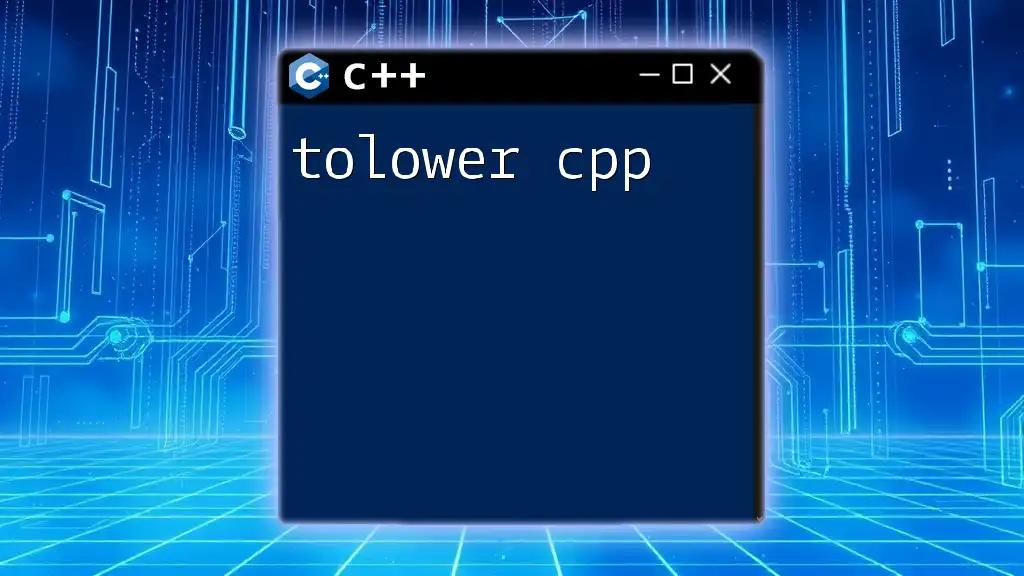
Interfacing R and C++
The ability to call C++ functions directly from R is one of the most powerful features of R cpp.
Calling C++ functions from R
Utilizing the `.Call()` interface from R allows seamless integration of C++ functions, enhancing performance without complicated setups.
Here's how you can call a C++ function that computes the mean of a numeric vector:
#include <Rcpp.h>
using namespace Rcpp;
// [[Rcpp::export]]
double cpp_mean(NumericVector vec) {
return mean(vec);
}
You can invoke this function in R the same way you invoked previous examples.
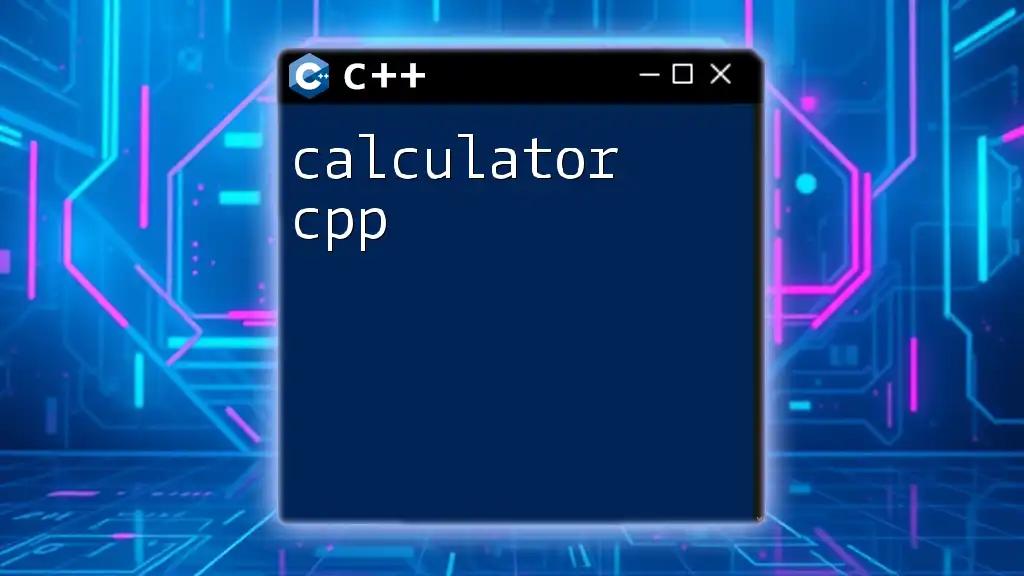
Performance Optimization with R cpp
One of the compelling reasons to adopt R cpp is to enhance your code's performance, especially with large datasets.
Why optimize R code with C++
R can manage data frames and statistics well, but as datasets grow, certain operations may become slow. By offloading computationally expensive tasks to C++, significant speed benefits can be observed.
Examples of optimizing R code using C++
As an example, consider optimizing a loop in R:
n <- 1e6
result <- 0
for (i in 1:n) {
result <- result + i
}
This R code can be inefficient for large `n`. Instead, you can replace it with an optimized C++ function:
#include <Rcpp.h>
using namespace Rcpp;
// [[Rcpp::export]]
double cpp_sequence_sum(int n) {
return n * (n + 1) / 2.0; // A formula for summing first n integers
}
This direct computation significantly reduces time complexity. Benchmarking such optimizations typically reveals impressive speed contrasts between R and C++ implementations.
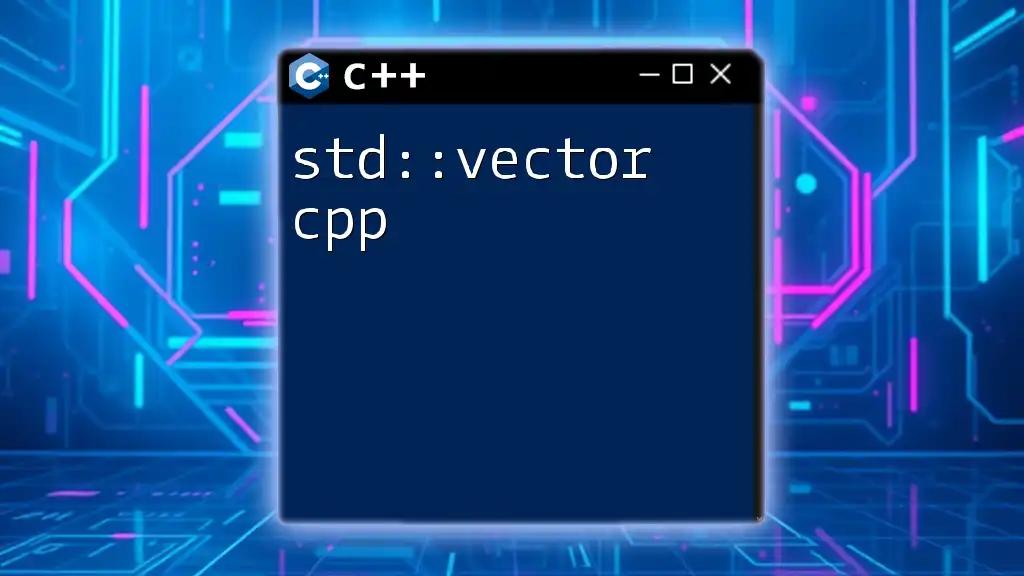
Debugging and Error Handling in R cpp
During development, you may encounter errors while interfacing R with C++. Knowing how to debug effectively can save you a lot of frustration.
Common errors and troubleshooting tips
Errors may arise due to mismatched data types or memory issues. Here are some common pitfalls:
- Forgetting to include `Rcpp` headers.
- Incorrect function signatures.
- Passing data types that are incompatible between C++ and R.
Using Rcpp's built-in debugging tools
Rcpp provides tools such as `Rcpp::stop()` for error handling. For example:
#include <Rcpp.h>
using namespace Rcpp;
// [[Rcpp::export]]
double safe_divide(double numerator, double denominator) {
if (denominator == 0) {
Rcpp::stop("Denominator cannot be zero"); // Error handling
}
return numerator / denominator;
}
This function checks for division by zero and triggers an error message, which helps in debugging.
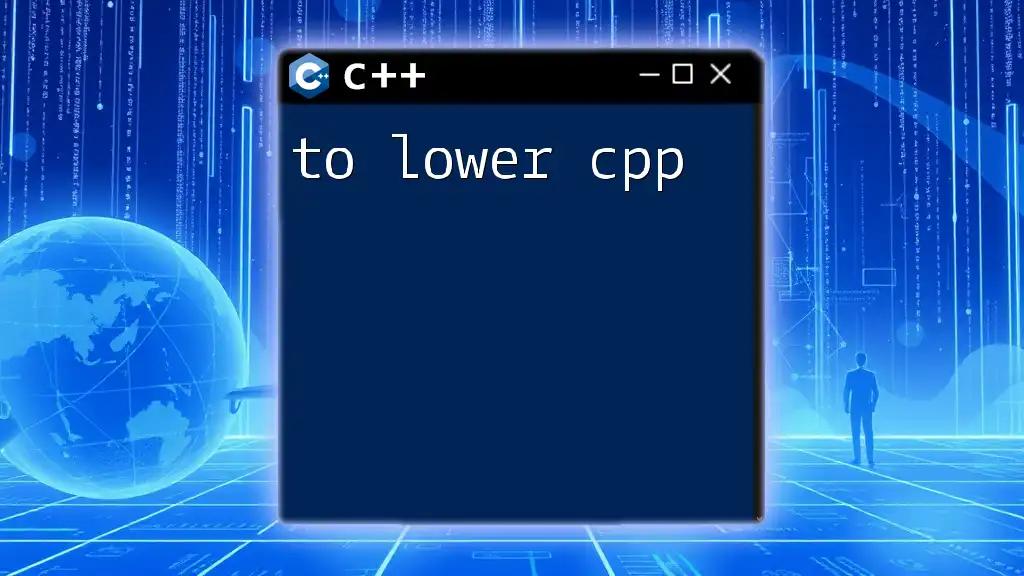
Advanced Topics in R cpp
If you're ready to take it a step further, exploring RcppArmadillo and RcppParallel can unlock powerful features.
Utilizing RcppArmadillo for linear algebra
RcppArmadillo is a C++ library for linear algebra, simplifying matrix operations. For example, matrix multiplication can be executed efficiently with minimal overhead.
First, install RcppArmadillo using:
install.packages("RcppArmadillo")
Then, try this linear algebra example:
#include <RcppArmadillo.h>
// [[Rcpp::depends(RcppArmadillo)]]
// [[Rcpp::export]]
arma::mat cpp_matrix_multiply(const arma::mat& A, const arma::mat& B) {
return A * B;
}
Incorporating RcppParallel for multithreading
Using RcppParallel can further enhance performance by taking advantage of multi-core processors. This is useful when processing large datasets.
Here is a simple example:
#include <RcppParallel.h>
// [[Rcpp::depends(RcppParallel)]]
using namespace RcppParallel;
// Create a class for our parallel task
class ParallelSum : public Worker {
// Input: matrix
const RMatrix<double> input;
// Output: Result matrix
RVector<double> output;
public:
ParallelSum(const double *input, double *output)
: input(input), output(output) {}
// Loop over rows
void operator()(std::size_t begin, std::size_t end) {
for (std::size_t i = begin; i < end; i++) {
output[i] = sum(input.row(i));
}
}
};
// [[Rcpp::export]]
RVector<double> cpp_parallel_sum(const RMatrix<double>& mat) {
// Result to store sums
RVector<double> result(mat.nrows());
ParallelSum sumTask(mat, result);
parallelFor(0, mat.nrows(), sumTask);
return result;
}
This function allows for the simultaneous summation of rows in a matrix, demonstrating the power of parallel processing.
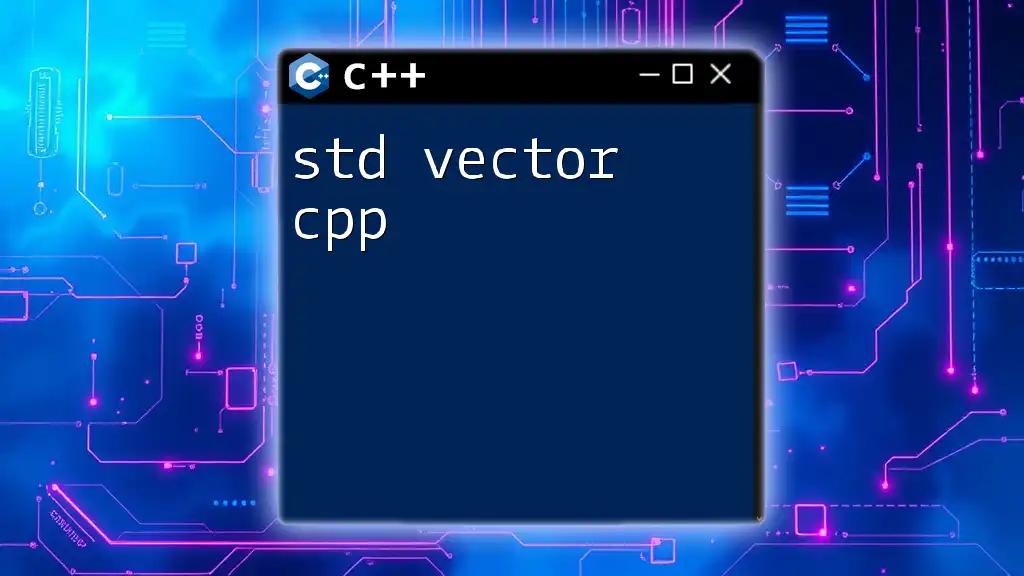
Best Practices for R cpp Development
When creating R cpp functions, it's vital to adhere to best practices to ensure your code is simple to maintain and scalable.
Tips for writing clean and maintainable code
- Organize your code by keeping function definitions tidy and well-documented.
- Use meaningful variable names that convey the purpose clearly.
- Comment on complex operations to aid future understanding.
Consider this example of a well-documented R cpp function:
#include <Rcpp.h>
using namespace Rcpp;
// [[Rcpp::export]]
// This function calculates the variance of a given numeric vector.
// The input is a NumericVector vec, and it returns a double value.
double cpp_variance(NumericVector vec) {
double mean = Rcpp::mean(vec);
double sum_sq_diff = 0;
for (double v : vec) {
sum_sq_diff += (v - mean) * (v - mean);
}
return sum_sq_diff / (vec.size() - 1);
}
Example: A well-documented R cpp function
Documentation not only assists other users but also aids you in recalling the purpose of functions down the line. Always include explanations of the parameters and return values.
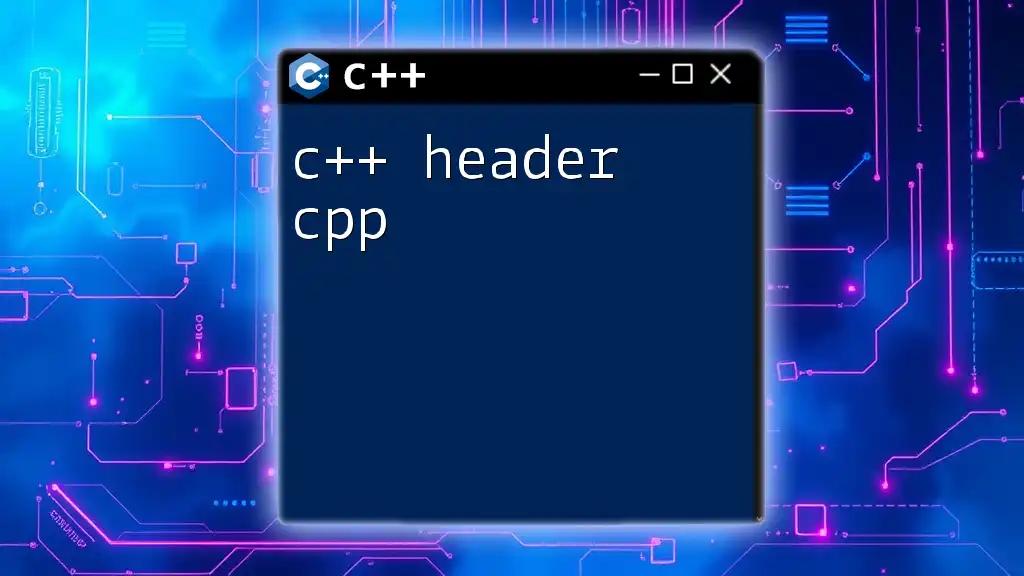
Next Steps and Resources
To take your R cpp skills to the next level, consider exploring additional resources:
- Books: "Rcpp: Seamless R and C++ Integration" by Dirk Eddelbuettel provides deep insights.
- Online Courses: Platforms like Coursera and edX offer courses specific to R and C++ integration.
- Community Forums: Join discussions on websites like Stack Overflow, where many engage in Rcpp-related topics.
In conclusion, R cpp is a powerful tool that combines the strengths of R and C++. By understanding its fundamentals, optimizing performance, and adhering to best coding practices, you can significantly enhance your data analysis capabilities. Happy coding, and enjoy your journey through R cpp!